【Pytorch】torch.narrowの引数・使い方を徹底解説!豊富なコード例を追加!
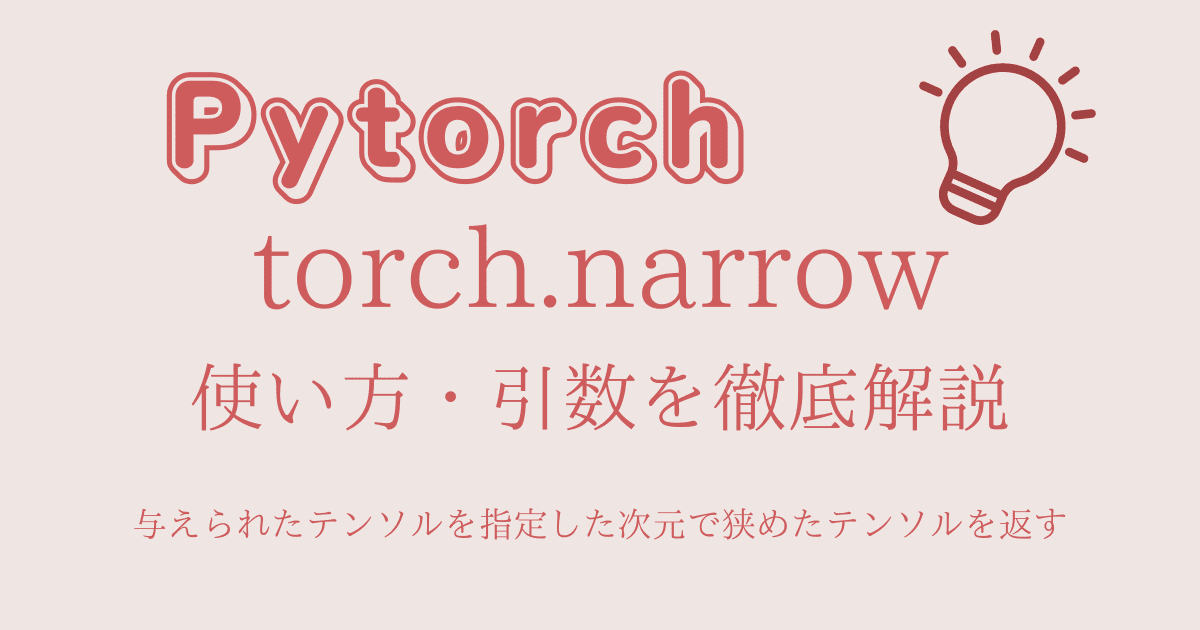
torch.narrowは与えられたテンソルを指定した次元で狭めた(一部の要素を取り出した)新しいテンソルを返す関数です。
目次
イメージを掴もう
イメージを掴むとtorch.narrowを扱いやすくなると思います。
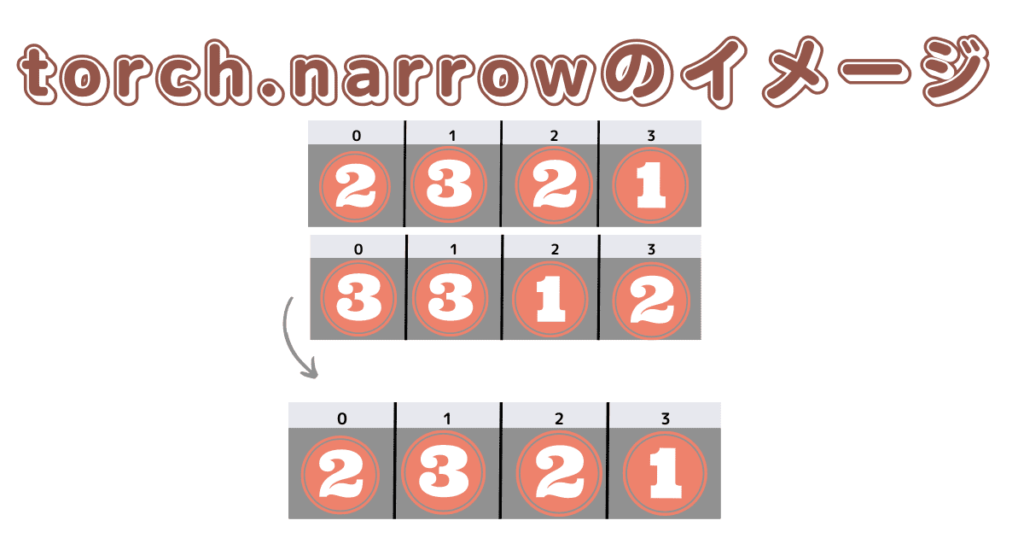
torch.narrowの引数
torch.narrowはこのように使うことができます。
import torch
# 入力テンソルの作成
input_tensor = torch.tensor([[1, 2, 3, 4],
[5, 6, 7, 8],
[9, 10, 11, 12]])
# 1番目の次元(列)を狭める
narrowed_tensor = torch.narrow(input_tensor, dim=0, start=1, length=2)
print(narrowed_tensor)
# tensor([[ 5, 6, 7, 8],
# [ 9, 10, 11, 12]])
では、torch.narrowの引数について見ていきます。
- input (Tensor)
狭める対象のテンソルです。この引数には、狭めたいテンソルを指定します。狭める操作はこのテンソル上で行われます。 - dim
狭める次元のインデックスを指定します。次元は0から始まることに注意してください。この引数には、狭めたい次元のインデックスを整数値で指定します。 - start (int or Tensor)
狭めた次元の開始位置のインデックスを指定します。負の値を指定すると、次元の末尾からのインデックスを意味します。また、この引数は整数値またはテンソルを受け入れますが、テンソルを指定する場合は0次元の整数テンソルである必要があります。テンソルを使用することで、複数の開始位置を一度に指定することも可能です。 - length (int)
狭めたい次元の長さを指定します。この引数はゼロ以上の値である必要があります。狭めたい次元の長さがlength
によって指定できます。
torch.narrowの注意点
dim
で指定した次元における長さは、start
+length
の長さを超えていなければいけません。
逆に、start+lengthの長さは、dimで指定した次元における長さ以下でないといけません。
この条件を満たさない場合は、RuntimeError: start (0) + length (6) exceeds dimension size (4).
のようなエラーが出ます。
dimの影響を理解する
dim=0
の場合
m = torch.arange(12).reshape(3, 4)
"""
tensor([[ 0, 1, 2, 3],
[ 4, 5, 6, 7],
[ 8, 9, 10, 11]])
"""
# dim=0, start=0, length=1
torch.narrow(m, 0, 0, 1)
"""
tensor([[0, 1, 2, 3]])
"""
# dim=0, start=0, length=2
torch.narrow(m, 0, 0, 2)
"""
tensor([[0, 1, 2, 3],
[4, 5, 6, 7]])
"""
# dim=0, start=1, length=2
torch.narrow(m, 0, 1, 2)
"""
tensor([[ 4, 5, 6, 7],
[ 8, 9, 10, 11]])
"""
length
は、start
基準でいくつに狭めるのかを設定するものだと分かります。
インデックスを指定している訳ではないので注意が必要ですね。
dim=1
の場合
m = torch.arange(12).reshape(3, 4)
"""
tensor([[ 0, 1, 2, 3],
[ 4, 5, 6, 7],
[ 8, 9, 10, 11]])
"""
# dim=1, start=0, length=1
torch.narrow(m, 1, 0, 1)
"""
tensor([[0],
[4],
[8]])
"""
# dim=1, start=0, length=2
torch.narrow(m, 1, 0, 2)
"""
tensor([[0, 1],
[4, 5],
[8, 9]])
"""
# dim=1, start=1, length=2
torch.narrow(m, 0, 1, 2)
"""
tensor([[ 1, 2],
[ 5, 6],
[ 9, 10]])
"""
dim=1の場合も同様にlength
は、start
基準でいくつに狭めるのかを設定するものだと分かります。
その他の引数の影響を理解する
コード例を色々と作っているので確認して見てください。
import torch
# 入力テンソルの作成
input_tensor = torch.tensor([[1, 2, 3],
[4, 5, 6],
[7, 8, 9]])
# 1番目の次元(列)を狭める
narrowed_tensor1 = torch.narrow(input_tensor, dim=0, start=0, length=2)
print("Narrowed Tensor 1:")
print(narrowed_tensor1)
# 出力:
# Narrowed Tensor 1:
# tensor([[1, 2, 3],
# [4, 5, 6]])
# 2番目の次元(行)を狭める
narrowed_tensor2 = torch.narrow(input_tensor, dim=1, start=1, length=2)
print("Narrowed Tensor 2:")
print(narrowed_tensor2)
# 出力:
# Narrowed Tensor 2:
# tensor([[2, 3],
# [5, 6],
# [8, 9]])
# 負の値を使用して次元を狭める(開始位置を末尾から指定)
narrowed_tensor3 = torch.narrow(input_tensor, dim=0, start=-2, length=2)
print("Narrowed Tensor 3:")
print(narrowed_tensor3)
# 出力:
# Narrowed Tensor 3:
# tensor([[4, 5, 6],
# [7, 8, 9]])
# Tensor型の開始位置を使用して次元を狭める
start_tensor = torch.tensor(1)
narrowed_tensor4 = torch.narrow(input_tensor, dim=1, start=start_tensor, length=2)
print("Narrowed Tensor 4:")
print(narrowed_tensor4)
# 出力:
# Narrowed Tensor 4:
# tensor([[2, 3],
# [5, 6],
# [8, 9]])
# 長さがゼロの場合
narrowed_tensor5 = torch.narrow(input_tensor, dim=0, start=0, length=0)
print("Narrowed Tensor 5:")
print(narrowed_tensor5)
# 出力:
# Narrowed Tensor 5:
# tensor([], size=(0, 3), dtype=torch.int64)
# 元のテンソルと狭めたテンソルの内容が共有されることを確認
input_tensor[0, 0] = 99
print("Original Tensor:")
print(input_tensor)
# 出力:
# Original Tensor:
# tensor([[99, 2, 3],
# [ 4, 5, 6],
# [ 7, 8, 9]])
print("Narrowed Tensor 1 (after modifying original tensor):")
print(narrowed_tensor1)
# 出力:
# Narrowed Tensor 1 (after modifying original tensor):
# tensor([[99, 2, 3],
# [ 4, 5, 6]])
まとめ
troch.narrow
は入力テンソルを狭めた新しいテンソルを返すstart
~start
+length
までを返す
あわせて読みたい
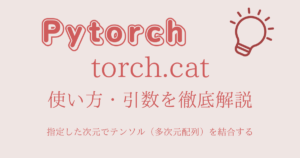
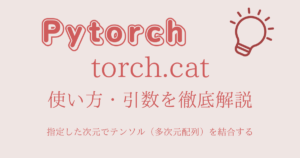
【Pytorch】torch.catの使い方・引数を徹底解説!テンソルを結合する方法
torch.catはPyTorchでテンソル(多次元配列)を結合するための関数です。この関数を使用すると、指定した次元でテンソルを連結することができます。 ドキュメント:torc...
あわせて読みたい
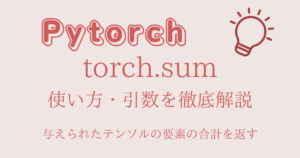
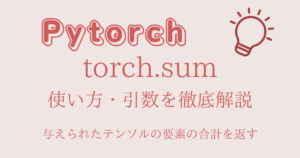
【Pytorch】torch.sumの使い方・引数を徹底解説!dim=-1, 0, (1, 1)などの意味とは?
torch.sumの役割は、与えられたテンソルの要素の合計を返すことです。 イメージを掴もう torch.sumの引数 引数は、torch.sum(input, dim, keepdim=False, *, dtype=None...
コメント